The increasing amount of data being processed in today’s electronic devices for classification tasks in image and audio recognition, autonomous driving, smart sensors signal processing or machine learning, requires a transition from the conventional compute centric paradigm to a more data centric paradigm. In the classical von Neumann architecture, the data is transferred between computing and memory units via a bus system with limited bandwidth, giving rise to the well-known von Neumann bottleneck. In order to bridge the existing gap between memory and logic units, the concept of physical separation between computing and memory unit has to be repealed.
Ferroelectric Tunnel Junction (FTJ) devices can be applied as synaptic weighting elements in neuromorphic processors, and offer the possibility of massive parallelism (fig. 1). These architectures use artificial neurons and synapses to emulate biological primitives underlying learning. In particular, the synapses act as both storage and computing element. Indeed, their role is to facilitate or inhibit the connection between neurons by changing their weight. In case of FTJs devices, the change of weight is mapped into a change of the device conductance.
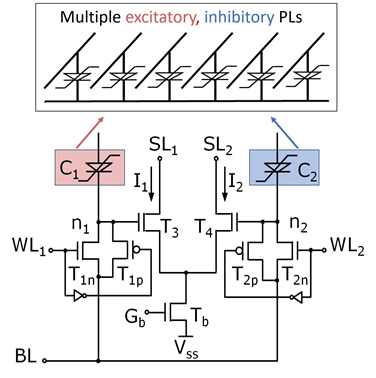
In order to use FTJ devices for applications in beyond-von Neumann computing, their properties should be further improved, for example increasing the tunneling current and the tunneling electroresistance ratio. BEOL-compatible processes can be developed to modify device behavior, such as work function engineering of the electrodes for increasing tunneling currents (fig. 2). Additionally, the device operation should be optimized to target specific functionalities and weight update schemes, which requires understanding of the device behaviour.
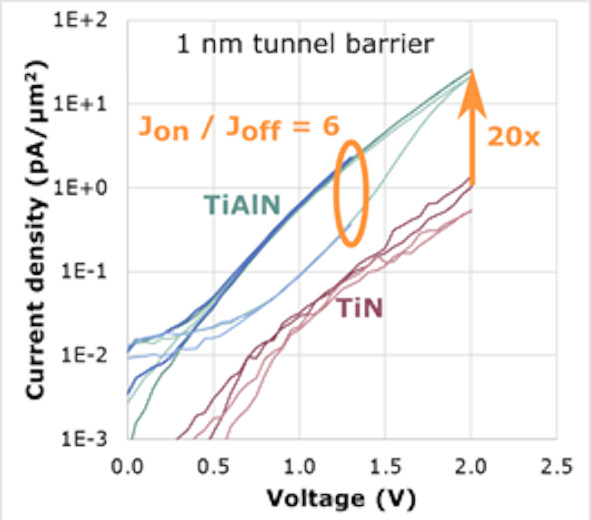
Novel devices based on spin-orbit interactions offer an alternative route to non-volatile memory devices. Skyrmions in magnetic bilayers can be used for memory-in-logic, with the benefit that they are topologically protected and highly robust. The integration of ferroelectric thin films with magnetic bilayers and device stacks designed for spin-orbitronics (fig. 3) enables investigation into a new generation of highly efficient, low-power, non-volatile components for beyond-von Neumann computing. The eventual target is to enable electric field control of magnetic spin textures.
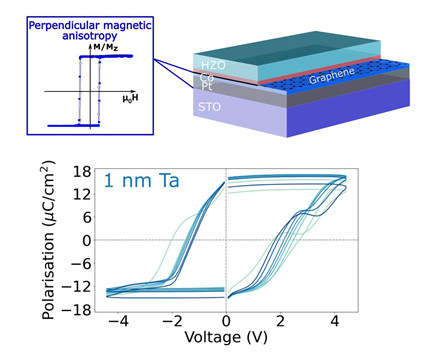
Cooperation:
X-FAB, IBM, IMDEA Nanociencia, CEA-Leti, CSIC, FAU, IUNET, Universities of Udine, Modena, Groningen, Zürich, HZB, NCSRD, ETH Zurich, AMAT, Melexis.